Monetizing AI/ML data has become a hot topic for enterprises, and rightly so: We appear at or near ‘peak AI’. It’s absolutely everywhere, and we’re even seeing companies like Dell put out expensive TV commercials talking about what they can do with things like generative AI.
Hype?
Some of it.
But a lot of it is real-world stuff about to explode on the real-world stage.
Notably, the word “explode” can have either a negative or a positive connotation, depending on the context.
AI is really the next generation of data analytics — a fancy new (although not really, more on that in a second) way to crunch data, ideally in true real-time fashion.
But companies are struggling to make the most of — ie, monetize — their AI/ML data. In fact, the overall return on AI projects has notably dismal thus far.
The fact is: AI and ML data have incredible business impact potential, but only with the support of true real-time data processing.
In this blog post, we’re going to try to cut through some of the hype to explain why and where companies are struggling the most to monetize their AI/ML data, and explore some strategies around how to face these challenges.
Table Of Contents
The AI Hype Cycle
The first thing we need to come to terms with is that “AI” goes through cycles — an important thing to consider for making realistic plans based on how things will end up, as opposed to how things are right now.
In 60’s and 70’s, “AI” as a concept existed only in cinema and TV, with the show Knight Rider featuring a sentient Pontiac Firebird and the deeply unbalanced “HAL 9000” stealing the show in the movie, “2001: A Space Odyssey.”
Why mention this in a tech blog about AI in 2024? Because our perceptions of what AI would be were heavily shaped by the media before any real AI existed. A lot of the frothy enthusiasm we are seeing now comes from the ideas fed to us when we were younger.
Machine learning is a statistical mechanism for tying inputs to outcomes that can be re-generated and updated as circumstances change. While it’s had an impact, it’s not been as much as the hype would have suggested.
Large language models (LLMs) are outwardly impressive but are ultimately limited by their need for historical training data that accurately represents not just the present, but the future.
So, assuming that we’ve taken the hype cycle into account and your AI/ML data idea is still a good one, what challenges will you face making money out of it?
Five Challenges With Monetizing AI/ML Data
1. Speed
Here at Volt, we deal quite often with companies whose applications need to make decisions in single-digit milliseconds because they are mission-critical. But, our prospective AI/ML customers (ie, the ones looking to start capitalizing on their AI/ML data) take longer, and generally make decisions in around 50 milliseconds. This is because AI engines are much, much slower than straightforward data manipulation. An awful lot of the available time will be spent cranking the handle of your AI decision engine, leaving little time for all the other stuff that has to happen.
When your business involves millions of small, fleeting chances to influence an event in the customer’s favor, or, in the case of something like credit card fraud prevention, stop an event from happening altogether, time is ‘of the essence’, and you may need everything to be very fast to make up for how long your AI decisions take.
The first major question you must therefore ask is:
Will this AI/ML-based application be fast enough to be useful?
An inability to respond fast enough to be relevant can be catastrophic for certain types of applications. Some companies only discover their speed (or performance) issues after building elaborate data and ML chains.
So if you’re in this boat with your applications, be sure to:
- Understand the needs of your audience as far as latency.
- Define hard expectations for response times, and
- Measuring the performance of prototypes before fully committing to going live.
2. TCO
The last thing you want is your ability to monetize your AI/ML data to be costly because then it’s like withdrawing two dollars for every dollar you deposit.
Data platform TCO is a growing issue.
It’s one thing to work in cases where a large amount of money is involved, such as a car purchase. But what if you’re using microtransactions to nudge people’s behavior in your favor or trying to optimize a process by 2-3%, which at a large scale could be worth millions, but at an individual event level almost nothing?
This is probably a good time to consider who owns and runs the ML/LLM in question, and what service-level agreements they offer. The entire LLM space is so volatile right now that significant and unexpected changes to what people are selling are more or less certain, and even a small price increase could cause major problems.
So the second major question is:
Will this project actually make money?
This one is both easy and hard.
The easy part is defining how much services will cost at launch time and defining the effectiveness rates they must hit to be commercially viable. The hard part is what assumptions you should make about how well this holds up in the future.
LLMs are an immature and volatile market, and it’s a well-established fact that many vendors are operating at a significant and unsustainable loss. The worst-case scenario is everything working perfectly until your LLM vendor ceases trading or doubles its costs, both of which are possible in the current market.
3. Accuracy
AI bots have a well-known problem with accuracy, with Air Canada recently losing a lawsuit after its bot gave bad advice.
Whether this is an issue or not depends on your individual scenario, but you should always consider that the users of these systems may have higher expectations for accuracy than you do, and accordingly they — and you — could face real-world consequences when errors occur.
So you have to ask:
Is your AI/ML-based application accurate enough, and can you weather the storm when it gets things wrong?
This means having your cake and eating it, too, (ie – not compromise on anything), but it could also mean choosing your battles very wisely.
In order to mitigate the risk of inaccurate information, you may need to significantly lower your expectations as to what areas you can use LLMs in, avoiding situations where you could be held liable for ‘hallucinations’.
4. Explainability
AI explainability is going to be a big issue, especially in the EU where the GDPR says that companies must tell consumers about:
the existence of automated decision-making, including profiling, referred to in Article 22(1) and (4) and, at least in those cases, meaningful information about the logic involved, as well as the significance and the envisaged consequences of such processing for the data subject.
Source: https://gdpr-info.eu/art-15-gdpr/
Given that LLM’s can’t explain why they took certain decisions, and thus can’t reveal the ‘logic involved’, this seems to be a major potential problem for the deployment of LLMs in the EU and other like-minded jurisdictions, as such decisions are indefensible in the eyes of the law.
Thus, the fourth question you should be asking is:
Will our plan survive contact with the GDPR? And if not, what do we do?
5. Making it “operational”
Operational challenges are the “Elephant in the room”.
Aside from all the issues above, remember that you will need to address a real-world, pragmatic issue:
Can you solve all of the four problems above at the same time in an integrated and reliable platform?
Here at Volt we have multiple customers who came to us after finding that solving all of these things simultaneously was near-impossible without a true real-time data processing platform that doesn’t force compromises.
Why Volt to Monetize Your AI/ML Data
At a time when so many people have their “Happy Ears” on for AI in general, and with LLMs especially, why ask the hard questions? Because we’ve seen too many AI projects, no matter how well-intentioned or brilliantly conceived, struggle to meet real-world expectations.
LLMs can’t makje you magically profitable any more than the invention of a better can opener will end world hunger. That said, under the right circumstances, and with the right input data, sane expectations, and favorable economics, they can definitely have a positive impact. But projects not backed by sound or proper data platform support are likely to struggle.
Volt has AI and ML data experts ready to help you fully capitalize on your AI and ML data. We work in the trenches, with your engineers, to help you get your AI and ML projects running smoothly and at the lowest possible cost.
Volt Active Data is the only data platform built to enable real-time data processing at scale for mission-critical applications. It’s a fast, scalable, accurate, data platform for taking real- time decisions so that you can get the most out of your AI/ML data.
If you can express your logic or decision-making process in Java, then you can do it in Volt, and even if you can’t, you can still use Volt to efficiently manage the interaction between a real-world event and your AI engine.
Contact us to learn more about how we enable enterprises to monetize their AI/ML data.
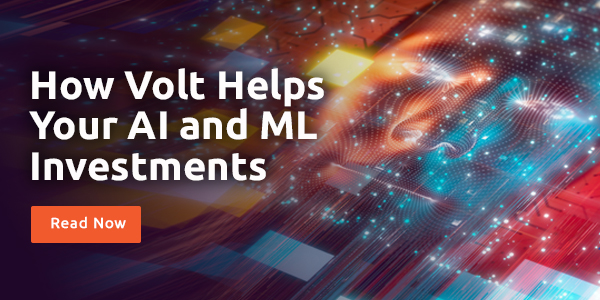